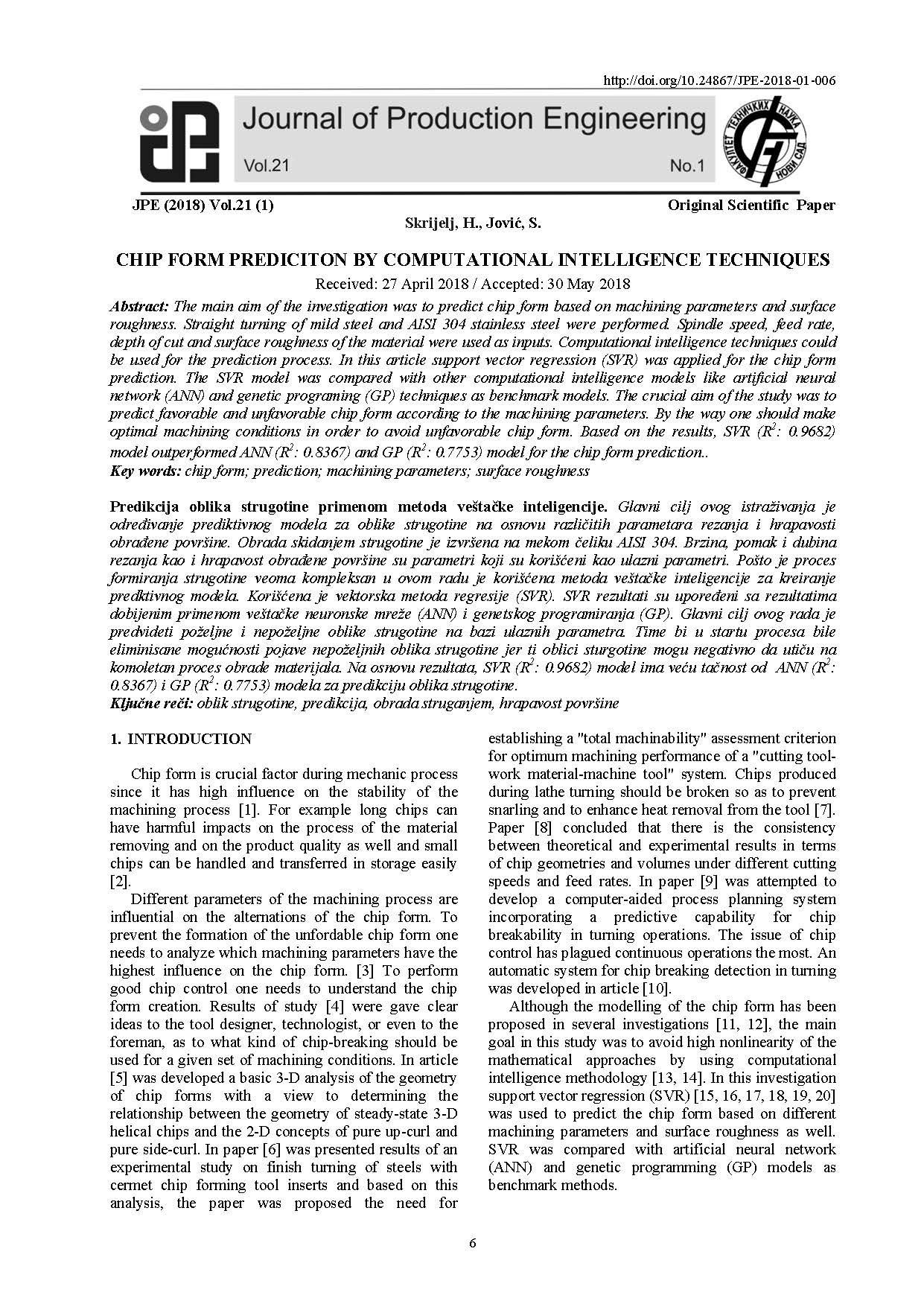
Published 2018-06-30
abstract views: 39 // FULL TEXT ARTICLE (PDF): 19
Keywords
- chip form,
- prediction,
- machining parameters,
- surface roughness
How to Cite
Copyright (c) 2023 Journal of Production Engineering

This work is licensed under a Creative Commons Attribution 4.0 International License.
Abstract
The main aim of the investigation was to predict chip form based on machining parameters and surface roughness. Straight turning of mild steel and AISI 304 stainless steel were performed. Spindle speed, feed rate, depth of cut and surface roughness of the material were used as inputs. Computational intelligence techniques could be used for the prediction process. In this article support vector regression (SVR) was applied for the chip form prediction. The SVR model was compared with other computational intelligence models like artificial neural network (ANN) and genetic programing (GP) techniques as benchmark models. The crucial aim of the study was to predict favorable and unfavorable chip form according to the machining parameters. By the way one should make optimal machining conditions in order to avoid unfavorable chip form. Based on the results, SVR (R2: 0.9682) model outperformed ANN (R2: 0.8367) and GP (R2: 0.7753) model for the chip form prediction.